Introduction
Fenwick tree, also known as binary indexed tree, is a tree that is indexed by the bits of its integer keys. It is usually useful data structure for implementing dynamic cumulative frequency tables. Usually, it can be used to answer Range Sum Query questions in a simpler fashion compared with segment tree. It is extremely efficient as they use fast bit manipulation techniques.
To explain how this works, we first define a function $LSOne(i)$, which produces the first Least Significant One-bit in $i$. The underlying operation is bit manipulation
(i & (-i))
.
A Fenwick tree is usually implemented as an array (a std::vector
in this blog), where indices fall in the range $[1 \ldots n]$. We will make the vector big enought to contain all the elements and also skip index zero for simplicity. Let’s assume that the Fenwick tree is ft
. Then the element at index $i$ is responsible for elements in the range $[i-LSOne(i)+1 \ldots i]$, that is from the index: $i$ subtracted by its least significant one-bit plus one, to the index: $i$.
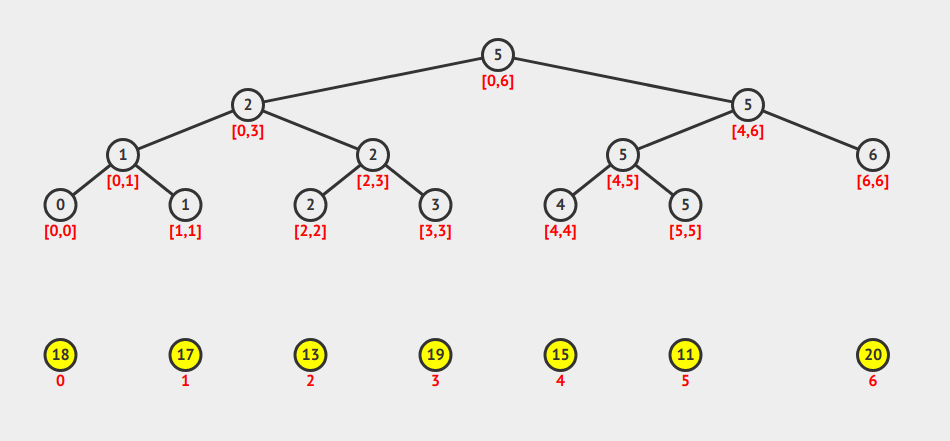
Operations
Once the tree is built, the next question is how to use it, dynamically. There are two basic operations: 1. query, 2. update. We will go through them in details.
Query
The first common operation is to query frequency from the start(1) to some index $b$. Namely, we call all query $rsq$. So the following is how we do a query $rsq(b)$:
- (Init Sum) Let $sum \leftarrow 0$
- (Accumulate Sum) $sum \leftarrow sum + ft[b]$
- (Get new $b$) $b \leftarrow b - LSOne(b)$
- (If ended?) If $b == 0$, return $sum$, otherwise goto step 2
With the above query available, obtaining the cumulative frequency between two indices $[a\ldots b]$ where $a != 1$ is simple, just evaluate $rsq(a, b) = rsq(b) - rsq(a-1)$.
Update (Adjust value)
Let’s name the operation of updating the value of the element at index $k$ by adjusting its value by $v$ to be $adjust(k, v)$. This is done by the following steps:
- (Adjust value at index $k$) $ft[k] += v$
- (Get new $k$) $k \leftarrow k + LSOne(k)$
- (If ended?) If $k < ft.size()$, goto step 1.
Implementation
FenwickTree Class
#ifndef FENWICK_TREE
#define FENWICK_TREE
#include <vector>
// Least Significant One-bit Macro
#define LSOne(i) (i & (-i))
class FenwickTree{
private:
std::vector<int> ft;
public:
FenwickTree(int n){ ft.assign(n+1, 0); }
// function to query range [1, b]
int rsq(int b){
int sum = 0;
for(; b; b -= LSOne(b)){
sum += ft[b];
}
return sum;
}
// function to query range [a, b]
int rsq(int a, int b){
return rsq(b) - (a == 1 ? 0 : rsq(a-1));
}
// adjusts value of the k-th element by v
void adjust(int k, int v){
for( ; k < (int)ft.size(); k += LSOne(k)){
ft[k] += v;
}
}
};
#endif
Test File
#include <iostream>
#include "./include/fenwick_tree.hpp"
#define LOG(X) std::cout << X << std::endl
int main(){
// assume we have following student scores
// ranging from 1 to 10
int f[] = {2, 4, 5, 5, 6, 6, 6, 7, 7, 8, 9};
FenwickTree ft(10);
// insert scores manually to an empty fenwick tree
for(int i = 0; i < 11 ; i++) ft.adjust(f[i], 1); // accumulate by one occurence
LOG(ft.rsq(1, 1));
LOG(ft.rsq(1, 2));
LOG(ft.rsq(1, 6));
LOG(ft.rsq(1, 10));
LOG(ft.rsq(3, 6));
ft.adjust(5, 2);
LOG(ft.rsq(1, 10));
}
Time Complexity
Because the building of a Fenwick Tree is manually done by calling
adjust(int k, int v)
function, so we first need to analyze the two basic
operations provided by Fenwick Tree. In query operation, an integer $b$ can at most have $O(\log b)$ bits. So the query operation has time complexity of $O(\log n)$ per-run.
Similarly for adjust operation, one change at index $k$ can at most affect $O(\log n)$ nodes in Fenwick tree.
With those in mind, the building of Fenwick Tree is clearly multiple runs of
adjust
function. So it takes time $O(m \log n)$ time to build such a tree, where
$m$ is the number of data points.
Leave a Comment